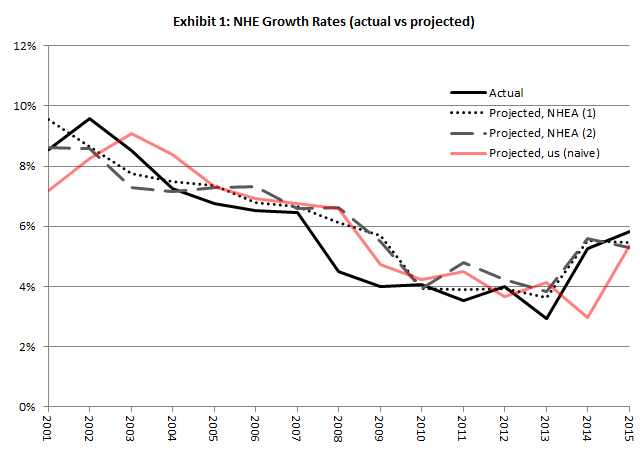
The health spending statistics and forecasts from the Centers for Medicare and Medicaid Services (CMS) Office of the Actuary are central to health policy evaluation. The current release, which provides actual numbers from 2015 and uses them to update projections for spending for the next decade, is no exception. Economist Tom Getzen has shown that these forecasts tend to be quite accurate, and have become more accurate over time. Since 1997 the mean absolute deviation (MAD) between forecast and actual growth in the first projected year is just 0.9 percentage points. Three years out, the MAD rises to 1.3 percentage points. (Mean absolute deviation is the average of the absolute value of the difference between forecasted and actual spending. Put more colloquially, it is the average size of the mistake.)
As good skeptical economists (i.e. curmudgeons), we wonder if this accuracy reflects superior modeling techniques or merely reflects a slow but predictable evolution of health spending. We decided to see how well we could do using a simple possible forecast based solely on the prior year’s growth. Before we report on how we did, some institutional detail is useful. First, bear in mind that the recent CMS release contains 2015 data that is used to predict spending that already happened in 2016 (i.e., we do not yet know how much we spent last year). Second, the forecasts are not adjusted for overall inflation, which fortunately has been quite tame for the past two decades. This helps explain the impressive recent performance of the CMS forecasts — since 2001 the MAD between the forecast and actual growth in the first projected year is just 0.6 percentage points. (Any differences between this and Tom Getzen’s earlier work are because we are only focusing a more recent time period.)
Our naïve forecasting model—based on a regression in which we forecast future spending based solely on prior year’s growth—also does well, with an MAD of 0.9 percentage points (note 1). Exhibit 1 provides a more detailed comparison among actual spending and the two forecasts. The Exhibit confirms that CMS bests our simplistic approach. But how much better is CMS doing?
Our MAD may be 50 percent larger, but the 0.3 percentage point difference is small relative to the 5.9 percent average spending growth. Perhaps, if we were to factor in basic macroeconomic conditions, such as labor market growth (which CMS largely ignores but we have previously shown affects both private and Medicare spending growth), our simple forecast would have equaled or outperformed CMS.
The Difficulties Of Accounting For Macroeconomic Trends
It is not our intention to play Monday morning quarterback and we are not here to criticize CMS. It is often difficult to outperform naïve forecasts based on trends, and predicting health care trends is no exception, as there are too many moving parts for anyone to substantially outpredict the trend line. Take, for instance, the relationship between the macro-economy and health care spending. Our prior research shows that abrupt changes in macroeconomic conditions will produce abrupt parallel changes in health spending. But predicting macroeconomic shocks is perhaps more perilous than predicting health spending. Moreover, it is difficult to be certain which aspects of the macroeconomy should form the basis of health spending forecasts. In most recessions, gross domestic product (GDP) is a good predictor of health spending. But in the last recession, GDP recovered quickly while labor market participation did not, and the latter proved to be a more reliable predictor of health spending. CMS could not have predicted the onset or depth of the Great Recession or foreseen changes in the relative usefulness of different macro-economic indicators.
Do The CMS Spending Forecasts Really Assume Unchanging Laws?
CMS has baked into their methods another obstacle to accurate forecasting by assuming that there will be no changes to current laws and regulations. CMS includes a caveat to this effect, stating, “The NHE projections are constructed using a current-law framework and thus do not assume potential legislative changes over the projection period, nor do they attempt to speculate on possible deviations from current law.” This is a strong assumption, especially in recent years.
We believe that this raises two important issues. The first is about whether this statement is, in fact an accurate description of the methodology. The second is about what this means for how the projections should be interpreted and whether they should be expected to be accurate.
Deep in the guts of a technical paper, CMS provides a more precise if less elegant explanation of its forecast methods:
The models used to project trends in health care spending are estimated based on historical relationships within the health sector, and between the health sector and macroeconomic variables. Accordingly, the spending projections assume that these relationships will remain consistent with history, except in those cases in which adjustments are explicitly specified.
In other words, CMS bases its forecasts on aggregate time series data. This stands in contrast with regression and similar models that are often used to evaluate specific policy changes and explicitly account for the behaviors of individuals and organizations. But the CMS approach does not actually assume that laws do not change. Instead, it assumes that the cumulative effect of (unanticipated) future law changes will resemble the cumulative change of past law changes.
Another way of looking at this is that CMS is assuming that the past performance of policymakers will in fact predict future performance. This may have served CMS well during the two term administrations of President Bush and President Obama, but given the new uncertainties about health policy under President Trump, this has become a heroic posture.
What does this mean for interpretation? For a moment, set aside access and quality as policy goals and suppose that all laws were designed to curb spending growth. If CMS projections were really based on a current law framework, we would expect them to consistently over-predict spending growth, because they would fail to incorporate longstanding legislative and regulatory efforts to curb spending growth. But the technical paper suggests that their projections bake in past trends in laws and regulations. If those trends have remained relatively constant—i.e., there is no pronounced change in the rate of introduction and effectiveness of new laws and regulations—then we would expect CMS to get spending forecasts right on average. Given that the projections are designed as a blend of the two, we are unfortunately left at a loss of what lessons we should be able to take away from whether spending is growing faster or slower than projected.
The Uncertain Effects Of Health Care Innovation
As a final example of the challenges that CMS faces in projecting spending growth, we note that innovations that may affect health spending are hard to predict. This combination leads to peaks and troughs in spending growth. For example, Exhibit 2 presents prescription drug spending growth over time — an area where innovation is especially important. Here, we see a noticeable difference in the accuracy of one- and two-year projections. For example, the two-year projections fail to capture the patent cliff that led to decreased drug spending growth rates during the 2000s. Similarly, the two year projections fail to capture the effect of Sovaldi in 2014.
CMS one-year projections are still quite accurate, but these are produced after the year in which innovation effects have been realized. Thus, for example, the one-year ahead projections for 2014 were produced well after Sovaldi came to market and well after a substantial amount of attention was drawn to the pricing and usage of Sovaldi. When it comes to these types of innovations, it is clear that CMS could do more to capture the likely effects. Clinical trial pipeline data and information about drugs awaiting approval from the Food and Drug Administration (FDA) are widely available, as are analyst reports about sales expectations. Explicitly including these in their modeling would increase accuracy.
Signs Of A Return To Historical Levels Of Spending Growth
We would be remiss not to comment on the information in the new CMS forecasts, especially the actual (as opposed to projected) rate of growth in spending in 2015. We now have two consecutive years in which health spending growth has rebounded to nearer to (though still below) historical levels. Perhaps this should be expected, given the massive increase in coverage under the Affordable Care Act (ACA). Given this fact, it could be that absent the expansion health spending growth would actually have been much lower.
However, the previous projection for 2014 was roughly what we saw in the hard data, which has led some to claim that the net effect of the ACA expansions has been effectively zero. For example, in a separate post, Sherry Glied argues that because spending in 2014 was no higher than was projected prior to the passage of the ACA, that the ACA had accomplished its coverage expansions at effectively no costs. Indeed, this seems to be one of the most promising uses of spending forecasts — compare actual to forecasted spending and if the former is lower, give credit to policymakers.
We are unwilling to take such a strong stand for two reasons. First, the effect of many policies, including coverage expansions, are likely to be small enough to be background noise against the overall uncertainty of long-term CMS forecasts. For example, if we extrapolate from the Oregon Health Experiment, the recent 5 percentage point increase in the Medicaid population would result in just a one-time 1.25 percentage point increase in health spending. This is on a par with the MAD of five-plus year forecasts as reported by Getzen. The fact that CMS forecasts nearly exactly equaled actual 2014 spending was mere happenstance, given the inherent noise.
Second, and more important, Glied’s assessment ignores other factors that may have affected health spending that did enter into CMS’ forecasts, notably the lingering effects of the Great Recession.
While Glied and others may be reading too much into the similarities between CMS forecasts and actual spending, we wonder if there is not more to be learned when CMS forecasts are wrong, such as in the years immediately after the Great Recession. Something affected the health care economy in those years that CMS missed. Such moments are a learning opportunity for all of us, and should command our attention.
Note 1
Specifically, to make a projection for year t, we run a regression of spending growth on lagged spending growth using data from t-20 to t-1 and then use that regression output to predict spending growth for year t. The results weren’t particularly sensitive to our time window. We thank Mohammad Zuhad Hai for doing this analysis.